Evolutionary systems and ALife
We develop and analyse evolutionary systems to advance the understanding of natural and artificial evolutionary processes.
Our earliest work on the neuroevolution of articulated virtual creatures produced the first realistic co-adapted behaviours to have evolved using general-purpose neurons to control 3D physically simulated agents. The previous need for ad hoc (problem-specific) neurons was a barrier to the long-term evolution of general behaviours. Our research in this area is now focused on the incremental neuroevolution of complex reactive and deliberative behaviour, coupled with motor control: evolving articulated virtual creatures capable of performing tasks that cannot be solved by reactive mechanisms alone and which would traditionally have their solutions formulated in terms of search-based planning.
Through EPSRC project EP/H031936/1 Evolution as an Information Dynamic System, we worked with partners from the Universities of Manchester, Middlesex and Warwick. Our work on mutation rate plasticity established optimal mutation rate control functions, for both artificial landscapes and natural landscapes defined by DNA-transcription factor affinities, and demonstrated general applicability to biology. The Evolutionary Systems Group used tables of DNA to protein binding affinities to evolve DNA sequences in computer simulations, and a meta-Genetic Algorithm to evolve fitness-dependent mutation rate mappings that show a close match with the research team's predictions from information theory. We used Nvidia's CUDA many-core (GPGPU) technology to speed up computational experiments from 1.4 years (maximum, per run) to 3 days, enabling a step change in the rate at which the research was able to advance. The team then had a follow-on BBSRC project BB/L009579/1 The theory and practice of evolvability: Effects and mechanisms of mutation rate plasticity. In this project we are primarily investigating the effects and mechanisms of density-dependent mutation rate plasticity, although we first extended our previous work to determine optimal mutation rate control under selection.
Through the above Evolution as an Information Dynamic System project, the team also investigated the relationship between mutation rate, genetic loss and population size. Above a critical mutation rate (CMR) fitter genotypes may be outcompeted by those with greater mutational robustness. Expressed in terms of fitness landscapes, narrow high fitness peaks may be lost by a population while broader, lower peaks are maintained; this phenomena is referred to as "survival of the flattest". We established that CMR has an exponential dependence on population size in haploid populations: that as population size falls, the CMR above which fitter alleles are lost transitions unexpectedly from near-constant (the previous assumption in evolutionary biology) to drop exponentially for small populations, leaving them spiralling toward extinction. We subsequently verified that our model closely reproduces the less significant but established mathematical relationship between population size and 'error threshold' (no mathematical model has yet been derived for CMR), and established that the result also holds for diploid populations. This and the team's related research led to our BBSRC project, BB/M021157/1 Adaptive landscapes of antibiotic resistance: population size and 'survival-of-the-flattest'. In this, the focus was on the small population of a microbe with a newly arisen antibiotic resistance mutation.
We recognise the importance of research-led teaching. Evolutionary Systems and Neural Networks are taught together in our undergraduate programmes, through Computational Intelligence modules embedded within our single and dual honours Computer Science programmes.
Please get in touch if you want to learn more about our on-going Evolutionary Systems and ALife research, our current projects or open positions in our lab.
Contact
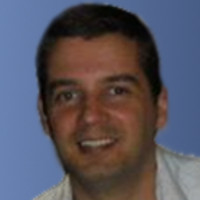
Dr Alastair Channon
Reader
Computer Science
- Colin Reeves, CR01
- +44 (0)1782 734270
- a.d.channon@keele.ac.uk